It’s 2022 and technology and smartphones or iPads are being used in multiple ways to help identify signs of autism for remote evaluations. This week’s blog discusses one of them, called CanvasDx which received FDA marketing authorization in June 2021.
By Lani Hessen, Sr. Director Patient Advocacy, Cognoa Inc[1] .
What are the current problems in autism evaluations and diagnosis?
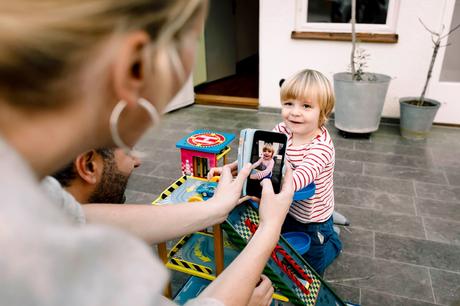
On average, parents first report concerns about their child’s development to their primary care provider when the child is 14 months old [1]. While parental concern is highly indicative of a potential autism spectrum disorder it is common for families to experience a prolonged wait before receiving their child’s diagnosis[2]. Delayed diagnosis can lead to missed opportunities to start ASD specific intervention during the early developmental period where it can have a greater benefit[2] than later intervention [3–8]. While a reliable ASD diagnosis can be made for a child as young as 18 months, the average age of diagnosis in the United States remains very high at 4 years and three months [9–11]. This age has remained largely unchanged for over 20 years despite increased awareness of the value of early intervention [9]. On average, caregivers report a three year delay between the time of their first concern and their child’s eventual diagnosis. Some children, such as girls, those who are non-White, or those who live in rural or remote areas can wait much longer than this [12–15].
Many factors contribute to diagnostic delays. ASD evaluations are usually conducted by specialists with ASD diagnostic expertise. Unfortunately, there are not enough of these specialists to meet the growing need for ASD assessments. As a result, many families end up on long wait-lists [16]. If primary care providers could assess and diagnose more children in the medical home, this could decrease strain on our specialty services and shorten wait-times. However, current diagnostic tools [17] are difficult to use in primary care settings; they take a long time to administer, are best conducted in-person, and may require specialist training [18]. Primary care providers require efficient, practical solutions that equip them to diagnose more children in the medical home. In the context of the ongoing COVID-19 pandemic, they also require clinically validated ASD diagnostic tools that can be delivered remotely.
What is Cognoa and can they help?
Cognoa is a pediatric behavioral health company developing diagnostic and therapeutic products with the goal of enabling equitable access to care and improving the lives and outcomes of children and families living with behavioral health conditions, starting with autism.
Cognoa’s diverse team of experts includes data scientists, engineers, researchers and clinicians. Our medical and advocacy team has expertise across a range of disciplines including occupational therapy, psychology, child and adolescent psychiatry, nursing, pediatrics, and developmental disability. We are committed to listening and learning from the voice of the community.
Did Cognoa talk to stakeholders while they were developing the tool?
We have spoken to families and heard them express frustration when primary care physicians do not take their concerns seriously. Additionally, families express concern about the lack of ASD specific education physicians receive. It often falls on caregivers to become the autism experts, and they may be left to coordinate their child’s care needs with minimal support. Navigating the diagnostic journey can be daunting due to conflicting advice, fragmented services and long wait-lists for specialist assessments. We have heard from families that they would welcome tools and research that could streamline this process. Additionally, self-advocates have expressed the need for a data driven approach that is inclusive of all presentations of ASD, thereby decreasing mis-diagnosis or delayed or missed diagnosis.
Primary care physicians have also expressed a number of concerns with the current diagnostic process including frustration over the length of time many families they refer are required to wait before receiving a specialist evaluation. Some primary care physicians have described lack of sufficient ASD specific training and tools as additional barriers to care. The primary care setting is also very time-pressured, and physicians have described how difficult it can be to carve out sufficient time to comprehensively evaluate, review results and discuss treatment plans with families [19,20].
How does this work? What problem does it solve?
The team at Cognoa has developed a digital diagnostic device called Canvas Dx to help address ongoing delays in ASD diagnosis and the lack of diagnostic tools available for primary care use. Canvas Dx is a prescription AI-based diagnosis aid intended to support primary care doctors to accurately and efficiently diagnose autism in children ages 18-72 months who are at risk for developmental delay based on a caregiver/parental or doctor concern. It falls under a class of products called software as a medical device (SaMD)[21], which includes software or mobile apps that are intended to treat, diagnose, monitor, mitigate, or prevent disease or other conditions.
Canvas Dx makes use of a machine learning algorithm that was initially developed using patient record data from thousands of children with diverse conditions, presentations, and comorbidities who were either diagnosed with ASD or confirmed not to have ASD based on standardized diagnostic tools and representing both genders across the supported age range. The Canvas Dx algorithm has been iteratively improved and prospectively validated over the past 6-7 years prior to the pivotal study.[22–27]. In order to use Canvas Dx you need a prescription from your care provider and access to a smart-phone. The following information is then collected:
- Parents/caregivers complete a questionnaire via the Canvas Dx caregiver-facing app and upload two short videos of their child. The questionnaire takes about 10 minutes to complete.
- These videos are scored for behavioral features of autism by trained video analysts.
- A doctor completes a questionnaire based on a visit with the parent/caregiver and child. The visit can be remote or in-person.
Canvas Dx uses AI to analyze these three inputs, and if the information is sufficient, it generates a result that the doctor uses as an aid to diagnose or rule out autism. Or it may result in the need for further evaluation The device is not intended for use as a stand-alone diagnostic device but to complement clinical expertise.
5. Who should be asking for a prescription for this?
Your primary care physician can prescribe Canvas Dx if they think it is suitable. It is designed to be used for children aged 18-72 months who are at risk for developmental delay based on a caregiver/parental or doctor concern.
Should I ask my doctor about it?
You can speak to your doctor about concerns that you have for your child’s development and start a conversation about next steps to get your concerns resolved.
What do I need to do?
Bring your child to well-child visits and express any concerns that you have about your child’s development.
What happens after I finish it? Who looks at it? What does it tell me?
Once all 3 inputs are complete, Canvas DX produces a report for your primary care provider to review with you. The report will provide one of three outputs: Positive ASD, Negative ASD, or No Result.
Further action can then be taken based on your care provider’s clinical judgment. For example, a positive result, combined with your provider’s own judgment, may prompt your pediatrician to make referrals to autism specific interventions in your area. A negative result, in combination with your provider’s clinical judgment, may prompt your pediatrician to begin to address your concerns via other avenues that are specific to the type of developmental delay that you are concerned about. Your provider may recommend further evaluation by a specialist.
Then what?
Your care provider, as well as local and national autism advocacy and education groups, like ASF, can help to guide you toward next steps no matter what the outcome of the test.
References:
1. Matheis M, Matson JL, Burns CO, Jiang X, Peters WJ, Moore M, de Back KA, Estabillo J. Factors related to parental age of first concern in toddlers with autism spectrum disorder. Developmental neurorehabilitation Taylor & Francis; 2017;20(4):228–235.
2. Christensen DL, Maenner MJ, Bilder D, Constantino JN, Daniels J, Durkin MS, Fitzgerald RT, Kurzius-Spencer M, Pettygrove SD, Robinson C. Prevalence and characteristics of autism spectrum disorder among children aged 4 years—early autism and developmental disabilities monitoring network, seven sites, United States, 2010, 2012, and 2014. MMWR Surveillance Summaries Centers for Disease Control and Prevention; 2019;68(2):1.
3. Flanagan HE, Perry A, Freeman NL. Effectiveness of large-scale community-based intensive behavioral intervention: A waitlist comparison study exploring outcomes and predictors. Research in Autism Spectrum Disorders Elsevier; 2012;6(2):673–682.
4. Smith T, Klorman R, Mruzek DW. Predicting Outcome of Community-Based Early Intensive Behavioral Intervention for Children with Autism. Journal of Abnormal Child Psychology 2015 Oct 1;43(7):1271–1282. [doi: 10.1007/s10802-015-0002-2]
5. Dawson G, Rogers S, Munson J, Smith M, Winter J, Greenson J, Donaldson A, Varley J. Randomized, Controlled Trial of an Intervention for Toddlers With Autism: The Early Start Denver Model. Pediatrics 2010 Jan 1;125(1):e17. [doi: 10.1542/peds.2009-0958]
6. Mazurek MO, Kanne SM, Miles JH. Predicting improvement in social–communication symptoms of autism spectrum disorders using retrospective treatment data. Research in Autism Spectrum Disorders 2012 Jan 1;6(1):535–545. [doi: 10.1016/j.rasd.2011.07.014]
7. MacDonald R, Parry-Cruwys D, Dupere S, Ahearn W. Assessing progress and outcome of early intensive behavioral intervention for toddlers with autism. Research in Developmental Disabilities 2014 Dec 1;35(12):3632–3644. [doi: 10.1016/j.ridd.2014.08.036]
8. Vivanti G, Dissanayake C, The Victorian ASELCC Team. Outcome for Children Receiving the Early Start Denver Model Before and After 48 Months. Journal of Autism and Developmental Disorders 2016 Jul 1;46(7):2441–2449. [doi: 10.1007/s10803-016-2777-6]
9. Maenner MJ, Shaw KA, Baio J. Prevalence of autism spectrum disorder among children aged 8 years—autism and developmental disabilities monitoring network, 11 sites, United States, 2016. MMWR Surveillance Summaries Centers for Disease Control and Prevention; 2020;69(4):1.
10. van’t Hof M, Tisseur C, van Berckelear-Onnes I, van Nieuwenhuyzen A, Daniels AM, Deen M, Hoek HW, Ester WA. Age at autism spectrum disorder diagnosis: A systematic review and meta-analysis from 2012 to 2019. Autism SAGE Publications Sage UK: London, England; 2021;25(4):862–873.
11. Pierce K, Gazestani VH, Bacon E, Barnes CC, Cha D, Nalabolu S, Lopez L, Moore A, Pence-Stophaeros S, Courchesne E. Evaluation of the diagnostic stability of the early autism spectrum disorder phenotype in the general population starting at 12 months. JAMA pediatrics American Medical Association; 2019;173(6):578–587.
12. Delobel-Ayoub M, Ehlinger V, Klapouszczak D, Maffre T, Raynaud J-P, Delpierre C, Arnaud C. Socioeconomic disparities and prevalence of autism spectrum disorders and intellectual disability. PloS one Public Library of Science San Francisco, CA USA; 2015;10(11):e0141964.
13. Oswald DP, Haworth SM, Mackenzie BK, Willis JH. Parental report of the diagnostic process and outcome: ASD compared with other developmental disabilities. Focus on Autism and Other Developmental Disabilities SAGE Publications Sage CA: Los Angeles, CA; 2017;32(2):152–160.
14. Wiggins LD, Durkin M, Esler A, Lee L-C, Zahorodny W, Rice C, Yeargin‐Allsopp M, Dowling NF, Hall‐Lande J, Morrier MJ. Disparities in documented diagnoses of autism spectrum disorder based on demographic, individual, and service factors. Autism Research Wiley Online Library; 2020;13(3):464–473.
15. Shattuck PT, Durkin M, Maenner M, Newschaffer C, Mandell DS, Wiggins L, Lee L-C, Rice C, Giarelli E, Kirby R, Baio J, Pinto-Martin J, Cuniff C. Timing of Identification Among Children With an Autism Spectrum Disorder: Findings From a Population-Based Surveillance Study. Journal of the American Academy of Child & Adolescent Psychiatry 2009 May 1;48(5):474–483. [doi: 10.1097/CHI.0b013e31819b3848]
16. Gordon-Lipkin E, Foster J, Peacock G. Whittling down the wait time: exploring models to minimize the delay from initial concern to diagnosis and treatment of autism spectrum disorder. Pediatric Clinics Elsevier; 2016;63(5):851–859.
17. Falkmer T, Anderson K, Falkmer M, Horlin C. Diagnostic procedures in autism spectrum disorders: a systematic literature review. European Child & Adolescent Psychiatry 2013 Jun 1;22(6):329–340. [doi: 10.1007/s00787-013-0375-0]
18. Kaufman NK. Rethinking “gold standards” and “best practices” in the assessment of autism. null Routledge; 2020 Aug 27;1–12. [doi: 10.1080/21622965.2020.1809414]
19. Fenikilé TS, Ellerbeck K, Filippi MK, Daley CM. Barriers to autism screening in family medicine practice: a qualitative study. Primary Health Care Research & Development 2014/11/04 ed Cambridge University Press; 2015;16(4):356–366. [doi: 10.1017/S1463423614000449]
20. Self TL, Parham DF, Rajagopalan J. Autism Spectrum Disorder Early Screening Practices: A Survey of Physicians. Communication Disorders Quarterly SAGE Publications Inc; 2015 Aug 1;36(4):195–207. [doi: 10.1177/1525740114560060]
21. FDA. Software as a Medical Device (SaMD) [Internet]. FDA; Available from: https://www.fda.gov/medical-devices/digital-health-center-excellence/software-medical-device-samd
22. Abbas H, Garberson F, Liu-Mayo S, Glover E, Wall DP. Multi-modular AI approach to streamline autism diagnosis in young children. Scientific reports Nature Publishing Group; 2020;10(1):1–8.
23. Abbas H, Garberson F, Glover E, Wall DP. Machine learning approach for early detection of autism by combining questionnaire and home video screening. Journal of the American Medical Informatics Association Oxford University Press; 2018;25(8):1000–1007.
24. Levy S, Duda M, Haber N, Wall DP. Sparsifying machine learning models identify stable subsets of predictive features for behavioral detection of autism. Molecular autism Springer; 2017;8(1):1–17.
25. Tariq Q, Daniels J, Schwartz JN, Washington P, Kalantarian H, Wall DP. Mobile detection of autism through machine learning on home video: A development and prospective validation study. PLoS medicine Public Library of Science San Francisco, CA USA; 2018;15(11):e1002705.
26. Wall DP, Dally R, Luyster R, Jung J-Y, DeLuca TF. Use of artificial intelligence to shorten the behavioral diagnosis of autism. Public Library of Science San Francisco, USA; 2012;
27. Kosmicki JA, Sochat V, Duda M, Wall DP. Searching for a minimal set of behaviors for autism detection through feature selection-based machine learning. Translational psychiatry Nature Publishing Group; 2015;5(2):e514–e514.
28. Cognoa. Cognoa Receives FDA Marketing Authorization for First-of-its-kind Autism Diagnosis Aid. 2021 Jun 2; Available from: https://cognoa.com/cognoa-receives-fda-marketing-authorization-for-first-of-its-kind-autism-diagnosis-aid/