Photo courtesy of iStockphoto.
To say that economic forecasting is inherently difficult is an understatement. The economy is a complex adaptive system, with billions of independent agents and countless relations and interconnections between them acting in a dynamic dancing ecology. The discovery of economic forces, theories, and correlations is a fruitful social scientific endeavor that has given us great insight into the workings of markets and money, but the problem of seeing into the future and anticipating the change in an evolving macroeconomic system confounds plenty of experts. In the article, The Disputability of Macroeconomic Knowledge, I describe the challenge that we have even finding agreement about the way economic systems work. Predicting long term trends, cycle turning points, and sudden discontinuities, will likely never become a perfect science.
My own crystal ball remains as cloudy as anyone’s when it comes to predicting the future of the U.S. economy. However, I do know a way to improve the accuracy of macroeconomic forecasting through the combination of multiple and diverse projections. It is fairly well established that combining multiple diverse forecasts or methods can improve average accuracy over time. The phenomenon was captured in James Surowiecki’s popular book, The Wisdom of Crowds: Why the Many Are Smarter Than the Few and How Collective Wisdom Shapes Business, Economies, Societies and Nations. Surowiecki provides compelling case studies, such as Sir Francis Dalton’s data from the 1906 West of England Fat Stock and Poultry Exhibition, in which 787 participants tried to guess weight of a steer. The mean of the crowd’s estimates was 1,197 lbs. while the actual weight was 1,198 lbs., an amazing outcome (Page “Wisdom of Crowds”). Combining multiple forecasts together to obtain one crowd prediction appears to have some promise. In fact, this leveraging of the crowd to make better forecasts is the impetus behind prediction markets.
Some Background on Predictive Diversity
In their detailed text, Forecasting: Practice and Process for Demand Management, Hans Levenbach and James P. Cleary highlight the benefits of combing and averaging forecasts. Professional forecasters have held competitions, such as the M1 and the M2, in which various methods and techniques were tested. In tests of the exponential smoothing method, using 1,001 times series during the M1 competition, it was discovered that taking a simple average improved accuracy compared to the best individual forecast. Although the M2 competition showed gains from simple averaging, the best individual forecasts still outperformed the crowd. The same logic that applies to portfolio diversity in investing applies to diversity in forecasting, it reduces the risk of putting all of your eggs in one basket (Levenbach, Cleary 545). It was from this text that I first learned the merits of forecast combination and began to apply this technique in my professional work to great utility.
In the Principles of Forecasting: A Handbook for Researchers and Practitioners, an indispensible resource for predictioneers compiled by J. Scott Armstrong, there is a chapter devoted to the principles of combining forecasts. These are sometimes referred to as composite or ensemble forecasts. Armstrong (419) writes, “Combing forecasts improves accuracy to the extent that component forecasts contain useful and independent information…There are two ways to generate independent forecasts. One is to analyze different data, and the other is to use different forecasting methods. The more that data and methods differ, the greater the expected improvement in accuracy compared to the average of the individual forecasts.”
Based on research and expertise the principles recommended by Armstrong are to use diverse data or methods, combine at least five forecasts, use formal procedures for combination to avoid judgmental biasing, apply equal weights unless there is good reasons to do otherwise, trim the highest and lowest forecasts before calculating the mean, and use past track records and domain knowledge to determine the weights if there is strong evidence that supports it. Armstrong (431) also calls attention to evidence that indicates that combining econometric forecasts improved upon the accuracy of the individual forecasts. This suggests there might be promise for my goal of forecasting macroeconomics.
In a series of Great Courses lectures from The Teaching Company called, The Hidden Factor: Why Thinking Differently is Your Greatest Asset, Scott E. Page of the University of Michigan spends some time on the benefits of forecast combination. In one lecture, aptly titled “The Wisdom of Crowds,” he demonstrates the Diversity Prediction Theorem and a mathematically sound proof that the crowd squared error of an ensemble of forecasts will necessarily equal the average individual squared errors of the forecasts minus the diversity of the forecasts. The major implication of the Diversity Prediction Theorem is that having diverse forecast models or predictions is just as important as having individual precision. In a subsequent lecture titled “The Diversity Prediction Theorem Times Three,” Page illustrates another excellent case study, the Netflix Prize. I don’t have the time to discuss it here, so I recommend checking out this Great Courses series.
Leveraging Diversity to Make Better Macroeconomic Forecasts
In the fourth quarter of 2011, as I was planning for the next year’s budgets, I needed a good estimate of the economic numbers for 2012. Nothing too fancy, just the annual forecasts of national GDP growth, CPI inflation, and the unemployment rate. Rather than waste precious time and resources building my own complex econometric model for use simply as high level inputs into my core forecasting interests I decided it was best to find some credible forecasts already in the public domain. The problem for me was choosing between the many conflicting forecasts available in the media and the internet.
Forecaster Roy Pearson provides several great resources in his article, “A Baker’s Dozen Free Sources of Economic Forecasts”, publishing in the Winter 2010 edition of Forecast: The International Journal of Applied Forecasting. The volume of forward economic projections on the web is overwhelming, so Pearson’s article is a helpful way to narrow the list down to a manageable repertoire of credible forecasts. He chose the recommended baker’s dozen under five principled criteria, the forecasts are free on the web, they are reliably updated on a regular basis, they contain numerical predictions, they provide projections for months, quarters, and years, and finally they include an explanation of the assumptions behind the models. Pearson’s selected crowd of forecasters includes U.S. and Canadian financial institutions, the Federal Reserve, the National Association of Realtors, neural net models from the private Financial Forecast Center, and Yale University’s Fair Model. I excluded these latter two sources, plus one of the U.S. financial institutions, from my model for technical reasons, leaving me with ten diverse forecasts of the same macroeconomic data points.
I calculated a simple average of ten forecasts from Mesirow Financial, Northern Trust, PNC, Wells Fargo, MFC Global - Manulife, BMO Capital Markets, ScotiaBank, Royal Bank of Canada, National Association of Realtors, and The Federal Reserve Bank of Philadelphia Survey of Professional Forecasters. This process generated a forecast for GDP growth of 2.26, a CPI inflation of 2.31, and an 8.9% unemployment rate. This ensemble of macroeconomics forecasts was fairly close to the mark, with actual GDP growth clocking in at 2.20, CPI inflation climbing 2.07, and unemployment dipping to 8.1% in 2012. In all three predictions the Diversity Prediction Theorem holds, which has to be the case given that it is enforced by a mathematical proof. A more difficult and less certain test is whether or not the ensemble beat all of the individual forecasts.
With the GDP prediction the crowd outperformed all of the individual forecasts, with the closest of those estimates being 2.1 and coming from both Northern Trust and ScotiaBank. Evaluating the CPI projection shows that the ensemble was outperformed by seven of the individual forecasts, a poor showing for diversity. A closer inspection of the CPI forecasts reveals that one forecast in particular, a prediction that prices would rise 4.1, from the National Association of Realtors, surely brought the average up too high. The evaluation of the unemployment forecasts is a bit of a different story, in that there was a bias to the high side with four models expecting rates above 9% and only one model coming anywhere close to the actual 8.1%. Because of this, the ensemble forecast for unemployment was too high at 8.9%. All in all a mixed bag.
I would have benefitted from following J. Scott Armstrong’s advice and observed the principle of trimming the mean by eliminating the minimum and maximum individual forecasts from the ensemble. When I backtest with the trimmed means I get forecasts of 2.20 for GDP, which is right on the money, and 2.10 for CPI, which is tied with the two best individual predictions. In the case of unemployment using a trimmed mean would have made things worse, because it would have eliminated a prediction of 8.0%, the number closest to the actual, and the combined forecast would have been 9.0% instead. Still a mixed bag, but an improvement in two out of three so I’ll take it.
Macroeconomic Forecasts for 2013 and 2014
All of my analysis above has to be taken for granted by the average reader, as I never made the macroeconomic predictions in my evaluation public prior to the period being forecast. Although, I have provided my method and source inputs so theoretically someone else should be able to recreate the same backcasts and test my claims. Nevertheless, backward analysis receives significant utility imparted to it from the value of its forward looking applicability. In this spirit I am sharing my best estimates of the U.S. macroeconomic outlook for the next two years, an ensemble that roughly mirrors the method I used to forecast 2012, although this time I used trimmed means for the GDP and CPI, plus I dropped the two highest unemployment forecasts to slightly correct for last year’s bias in the models. Below are forecast for GDP, CPI, and unemployment for 2013 and 2014.
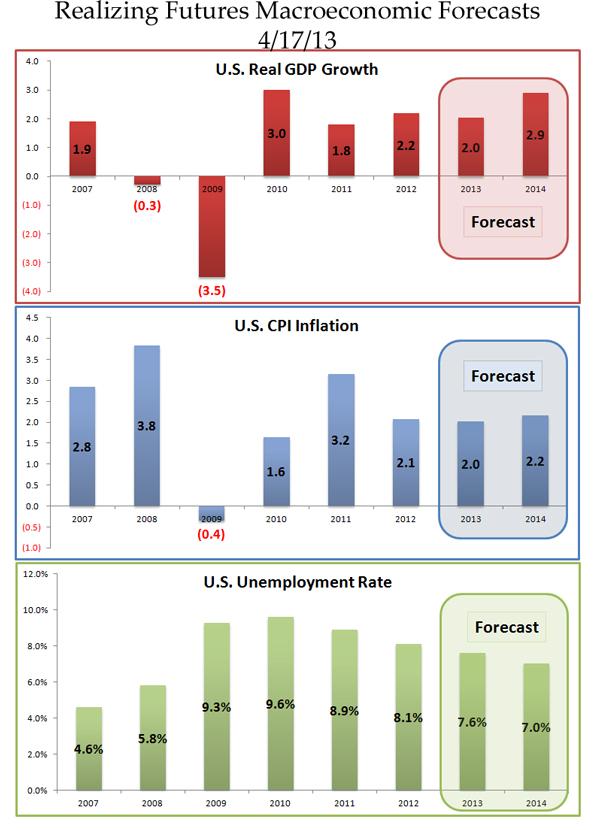
In the future I might be able to make further improvements to my ensemble model by weighting the input forecasts with unequal weights based on past accuracy or by careful scrutiny of the underlying model assumptions against domain knowledge (Levenbach, Cleary 545) (Armstrong). This is challenging because we don’t just want to pick the predictor that has done the best in the past because this removes the diversity benefit altogether, so selecting which forecasts to include and how to weight them can be a complicated optimization problem in which weights are assigned based on proportional accuracy or other scheme (Page “The Weighting is the Hardest Part”). I could also average forecasts taken at different lead times, seek out even more diverse models or forecasts to incorporate into my ensemble, or even build my own macroeconomic model as a supplement. Before going to all of this additional effort though it is helpful to consider whether the benefits in accuracy will outweigh the increasing costs of tracking and analyzing multiple models and methods.
Conclusion
Combining forecasts is not a panacea for perfect prediction. The economic system is far too complex and dynamic to provide consistent patterns and smooth trends all the time. Discontinuous changes, wildcards, sudden inflection points, and black swan events with low probability yet large consequence, are all facts of the global economic system. Disruptions can be dramatic and missed entirely by a consensus of astute experts, so averaging their predictions does not help in these scenarios. If we liken the situation to that of risk management in investing, as Levenbach and Cleary indicate, the comparison can be made to the way a diversified portfolio can hedge against idiosyncratic risk but not systemic risk. The same is true of forecasting with diverse models, the technique improves upon uncorrelated idiosyncrasies in different models or data sets, but it is not a hedge against something big and unexpected happening which is absent from all of the models. There is no silver bullet that will give us perfect knowledge of the future of complex systems. Still, by synthesizing and leveraging diverse predictive methods and models we can certainly enhance our predictive power and obtain better results than otherwise.
Jared Roy Endicott

Works Cited
Armstrong, J. Scott.. “Combining Forecasts”. Principles of Forecasting: A Handbook for Researchers and Practitioners. Ed. J Scott Armstrong. Philadelphia: Springer, 2001. 417-439. Print.
Levenbach, Hans, and James P. Cleary. Forecasting: Practice and Process for Demand Management. Belmont, CA: Duxbury, Thomson Brooks/Cole, 2006. Print.
Page, Scott E.. “The Wisdom of Crowds”.The Hidden Factor: Why Thinking Differently is Your Greatest Asset. Chantilly, Virginia: The Great Courses, The Teaching Company, 2012. Video.
Page, Scott E.. “The Diversity Prediction Theorem Times Three”.The Hidden Factor: Why Thinking Differently is Your Greatest Asset. Chantilly, Virginia: The Great Courses, The Teaching Company, 2012. Video.
Page, Scott E.. “The Weighting is the Hardest Part”.The Hidden Factor: Why Thinking Differently is Your Greatest Asset. Chantilly, Virginia: The Great Courses, The Teaching Company, 2012. Video.
Pearson, Roy (2010). “A Baker’s Dozen Free Sources of Economic Forecasts.” Foresight: The International Journal of Applied Forecasting, 16, 12-15.