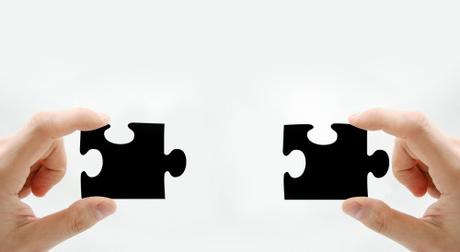
Lay users sometimes refer to voice interfaces as “speech recognition.” However, true speech recognition (converting sound waves into text representing spoken words), while an impressive technological feat, is but a stepping stone to unlocking the full potential of voice. It is the addition of a knowledge graph, robust natural language understanding models, and machine learning that truly enables the deployment of intelligent voice applications.
Definitions of “intelligence” generally comprise a capacity for logic, abstract thought, and continual learning. Going by this, an automated customer support line driven by pre-programmed voice commands is more sophisticated, but not necessarily more intelligent, than one reliant on keypad input. Similarly, there is a marked difference between a system that can be told to “turn on the lights” and one that can be asked an unfamiliar question like “are any kid-friendly Italian restaurants open right now?”
Today’s applications demand greater intelligence in understanding users’ naturally spoken queries. Diverse platforms – wristwatches, thermostats, Levi’s – are gaining connectivity without possessing a sizable screen, or even a screen at all, yet consumers expect the same high level of functionality that they’re accustomed to from mobile apps and websites. As technology becomes more deeply embedded in all aspects of life, it gets necessary to confront former barriers to access (such as hands or vision being otherwise occupied) without compromising the quality of the interaction.
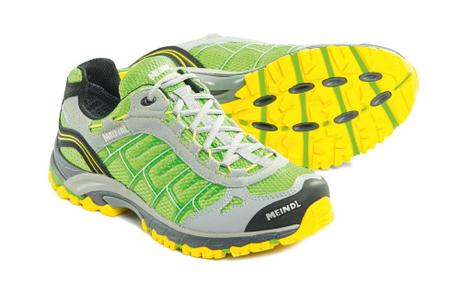
Recognizing words is not the same thing as recognizing meaning. Imagine you’re at a shoe store, trying on a pair of light green running shoes. If you tell the salesperson “let me try these in pink,” that person will grab the right pair from the back without a problem, even if the shoes are called “magenta” in the store catalog. While simple for a human, this task requires significant intelligence from a shopping app: for starters, “these” must be understood as the currently selected pair, and “in pink” must be understood as a color attribute matching “magenta.” If you were given results based on keywords alone, you would find looking at a pair of pink sandals, or perhaps even products from Victoria’s Secret’s Pink line.
Essentially, when a system determines which words were spoken and stops there, the user is only taken part way through the journey. “Speech recognition” does not entail a satisfactory voice experience on its own. To receive appropriate responses to unfamiliar broad-vocabulary queries, additional requirements must be met. This is where MindMeld comes in.
MindMeld’s advanced AI and NLP technology is designed to complement the great speech recognition capabilities built into all of the major OS platforms. When given a natural language query, MindMeld performs a syntactic analysis by identifying the key concepts and attributes and how they interrelate. Meaning is derived from a knowledge graph specifically tailored to an app’s domain (e.g. a store’s product catalog). This knowledge graph is filled with information on up to millions of different concepts, equipping apps with the ability to pinpoint true user intent and deliver results with speed and precision. Without such technology, the next generation of apps remains a sci-fi fantasy. With it, humans and devices will communicate more fluidly than ever before, enabling a future we can only begin to imagine.