In the realm of data analysis and visualization, Tableau stands as one of the most popular and user-friendly tools available. Among its many features, Tableau offers the capability to create forecasts, allowing users to predict future trends and make data-driven decisions. However, like any tool, Tableau has its limitations when it comes to forecasting. In this article, we'll explore these limitations, from data quality concerns to performance issues, and provide insights into how to work around them.
Before diving into the limitations of forecasting in Tableau, let's establish the context. Forecasting, in the context of Tableau, refers to the process of using historical data to predict future values. This is a crucial aspect of data analysis as it enables businesses to make informed decisions and plan for the future. Understanding the limitations is essential for accurate and effective forecasting.
What is Forecasting in Tableau?
Forecasting in Tableau refers to the capability of using historical data to predict future values, trends, or patterns within a dataset. It allows users to create forecasts and visualize them in interactive dashboards and charts, enabling data-driven decision-making. Tableau's forecasting functionality employs various algorithms, such as exponential smoothing and ARIMA, to generate predictions based on the provided data. Users can utilize these forecasts to gain insights into potential future developments, plan resource allocation, and make informed strategic choices, making it a valuable tool for businesses and analysts seeking to anticipate and respond to emerging trends and changes.
Limitations of Forecasting in Tableau
Tableau, a robust platform for data analysis and visualization, has its own set of limitations when it comes to forecasting. These limitations encompass data quality, model complexity, time-series analysis, visualization, performance, and user experience. In this article, we'll dissect each of these areas to offer a comprehensive view of the challenges users might face when utilizing Tableau for predictive analytics.
Data Quality Limitations
One of the fundamental limitations of forecasting in Tableau is its sensitivity to data accuracy. Inaccurate or incomplete data can lead to unreliable forecasts. Tableau's forecasting capabilities heavily rely on the quality of the input data. Garbage in, garbage out - a familiar mantra in the data world - holds true here. To address this, it's crucial to ensure data accuracy through rigorous data cleaning and validation processes before utilizing Tableau's forecasting functions.
Another data-related limitation lies in Tableau's ability to handle missing data. Missing values in your dataset can significantly impact forecast accuracy. While Tableau offers some tools to address missing data, it may not handle complex scenarios as effectively as specialized forecasting software.
Model Complexity Limitations
Tableau provides several forecasting algorithms, such as exponential smoothing and ARIMA, but it's essential to note that these options are limited compared to dedicated forecasting software. Users looking for advanced modeling options or specialized algorithms may find Tableau lacking.
While Tableau's forecasting functions are user-friendly, they come with constraints in terms of model customization. Advanced users might find themselves restricted when trying to fine-tune model parameters or incorporate domain-specific knowledge into the forecasting process.
Time-Series Limitations
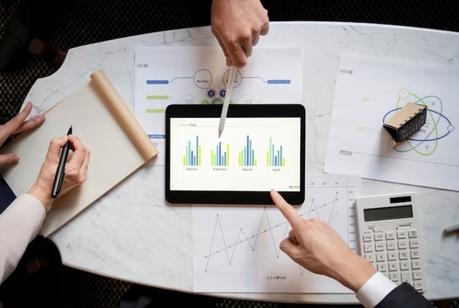
Seasonality is a critical component of many time-series data sets. Detecting and accommodating seasonality can be challenging within Tableau, which might affect forecast accuracy in industries where seasonality plays a significant role (e.g., retail, tourism, and agriculture). Tableau's forecasting capabilities may also pose limitations on forecasting far into the future. It excels at short-term forecasting but might not be suitable for long-term projections.
Visualization Limitations
Understanding the components of a forecast, such as trends and seasonality, can be challenging within Tableau. Users might find it difficult to gain deep insights into the underlying patterns driving the forecast.
While Tableau allows users to create multiple forecasts on a single chart, it can lead to visual clutter and reduced interpretability, especially when dealing with complex data sets.
Performance Limitations
Complex forecasting tasks can be computationally intensive, potentially straining Tableau's performance. Users working with large datasets or intricate forecasting models should be aware of possible slowdowns.
For organizations dealing with big data, Tableau's forecasting capabilities may not scale effectively. Handling massive datasets can be resource-intensive and may require additional optimization.
User Experience Limitations
While Tableau is known for its user-friendly interface, configuring forecasts can still be challenging for non-technical users. The learning curve might be steeper for advanced forecasting features. Tableau's forecasting functions may require manual intervention for tasks like model selection and evaluation. This can be time-consuming for users dealing with frequent forecasting updates.
How Much Data Needed for Forecasting in Tableau?
The amount of data needed for effective forecasting in Tableau can vary depending on the complexity of the forecast, the type of data, and the specific forecasting algorithm used. In general, a larger dataset with a rich historical context will often yield more accurate forecasts. However, there is no fixed rule for the minimum amount of data required, as it depends on factors like the level of seasonality, the volatility of the data, and the desired forecast horizon.
In some cases, even a relatively small dataset can be sufficient for short-term forecasts, while long-term or more complex predictions may require larger datasets spanning several years. It's crucial to consider the specific characteristics of your data and the forecasting goals when determining the adequate amount of data for Tableau forecasting.
Conclusion
In conclusion, Tableau is a valuable tool for data analysis and visualization, including forecasting. However, it's not without its limitations. Users should be aware of these limitations and consider alternative tools or strategies when dealing with complex forecasting tasks. While Tableau has its drawbacks, it remains a powerful asset in the data analyst's toolkit, especially for those who prioritize user-friendly interfaces and data visualization capabilities.
Understanding these limitations is the first step toward making informed decisions and achieving accurate forecasts in Tableau. By doing so, users can harness the strengths of Tableau while mitigating its weaknesses and disadvantages, ultimately enhancing their data-driven decision-making processes.