“What if Machine Learning could help to eradicate racial inequality in medicine?”
In a previous blog post, we explored problems with machine learning. We will continue to look at the use of algorithms and some problems that urgently need new approaches.
Analysis of algorithms widely used in US hospitals found that there has been systematic discrimination against black people. Researchers have identified 13 areas of algorithms that use race as a factor. It is suggested that it is vital that researchers and clinicians:
” . . . distinguish between the use of race in descriptive statistics, where it plays a vital role in epidemiologic analyses, and in prescriptive clinical guidelines, where it can exacerbate inequities.”
The use of medical algorithms to allocate health care to patients has led to Black people being less likely to be referred to programmers that aimed to improve care for patients who had complex medical needs.
Genetic predispositions
The problem is that there is substantial evidence that race—a social construct—is not a reliable proxy for genetics. Race is a too limited category and at the same time too broad. Thus, great care and knowledge are required when designing algorithms.
Admittedly, it is true that some populations are genetically predisposed to certain medical conditions, yet, these examples are rare. Genetic predispositions such as BRCA mutations (linked to breast cancer and more frequent among people of Ashkenazi Jewish heritage) simply do not apply to broad categories like “black” or “white”. There is a lot of diversity within each racial group and the problem is that biased algorithms end up making all the biases that we have in our health systems continue indefinitely.
What is the problem?
Biased medical algorithms have resulted in people who self-identified as black having been assigned lower risk scores compared to equally ill white people. This resulted in that black people were less likely to be referred to programmes that for example provided more personalized care. In one study, researchers found that the medical algorithms assigned risk scores based on total health care costs that the person accused in one year. It was assumed that higher health care costs are generally linked with greater health needs. Yet, a closer examination of the data found that the average black person was substantially sicker than the average white person. The amount of money someone spends on health care is not in itself a race-blind metric. Black patients access health care less than white patients and wealthier patients. By using cost as a metric the algorithm wrong concluded that black people were healthier than white patients since they spent less money on health care.
The problem is not one algorithm instead there is a problem with how entire health systems approaches the problem. So it is not a straightforward process to fix biases in algorithms. From a technical point of view, it is easy to rerun the algorithm using another variable the problem is the bias and injustices that are inherent and part of the society. How do you work around these biases?
Ways to overcome the biases
A problem is the lack of diversity among algorithm designers and an overall lack of understanding of the social as well as the historical context of the work.
The company Theator uses AI and computer vision to develop a surgical decision-making platform. Visual AI is used to scan video footage of real-world procedures and the system uses key moments during the surgery and provides notes about the situation. The idea is to create an intelligent, indexed library that provides the surgeons with insights into their performance. A study had shown that black children were three times more likely to die or experience complications after surgery than white children. The CEO of Theator believes that one way to close this gap is to use as much real-time data as possible.
Four types of machine learning algorithms
The ML algorithms can be broadly classified into four types:
- supervised,
- semi-supervised,
- unsupervised,
- reinforcement Machine Learning Algorithms
In the next blog post, we will look at these four types.
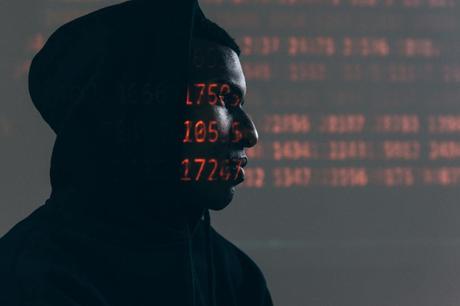