This little historical overview by recently completed undergraduate student, Sofie Costin (soon to join our lab!), nicely summarises the history, strengths, and limitations of species distribution modelling in ecology, conservation and restoration. I thought it would be an excellent resource for those who are just entering the world of species distribution models.
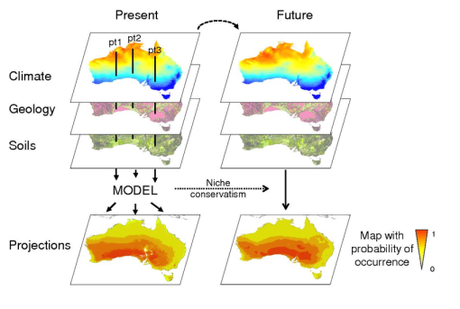
Of course, there is a strong association between and given species and its environment1. As such, climate and geographical factors have been often used to explain the distribution of plant and animal species around the world.
Predictive ecological models, otherwise known as ‘niche models’ or ‘species distribution models’ have become a widely used tool for the planning of conservation strategies such as pest management and translocations2-5. In short, species distribution models assess the relationship between environmental conditions and species’ occurrences, and then can estimate the spatial distribution of habitats suited to the study species outside of the sampling area3,6.
While the application of species distribution models can reduce the time and cost associated with conservation research, and conservation managers are relying increasingly on them to inform their conservation strategies4, species distribution models are by no means a one-stop solution to all conservation issues.Variables such as species range size2, sample size4,7, and sample bias8 all affect the reliability of the model projections, and a variety of model types have been developed with these limitations in mind7,9. It is therefore imperative that conservation managers using species distribution models to guide their actions
- ensure they are using the method most suited to the data they have available, and
- assess the accuracy of models before acting on the results9.
History
Species distribution models are widely used across marine, freshwater, and terrestrial applications10. They are most commonly used to predict the spread of pest organisms and to identify suitable habitats for the translocations of species outside the species’ current range11.
With advances in technology, including improved statistical techniques and geographical information systems (GIS), new and improved modelling methods9,12 have been developed. The complexity of these models has increased over time, from the matching of simple environmental variables, to fitting non-linear relationships between species’ presence and environmental, ecological, and climate variables 9.
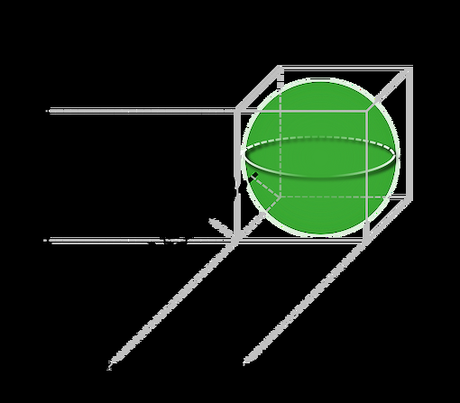
Multiple regression and generalised multiple regression are popular methods of modelling species distributions, and neural networks, locally weighted approaches, and environmental envelope models are also commonly used12. Additionally, weighted ensemble models (e.g., BIOMOD2) created from a combination of the aforementioned processes have been developed to increase model accuracy5. Machine-learning techniques are also on the rise and it is likely that new, more accurate methods with a wider range of applications will continue to be developed9.
Strengths and limitations
Species distribution models can provide a useful, reliable, and cost-effective method of estimating species distribution10.
But the selection of a suitable modelling method is essential to ensure that the predictions provide a realistic representation of real-world phenomena10,12. Discrepancies in predictive performance between modelling techniques are large, making it difficult for researchers without extensive modelling experience to choose the most reliable model for their needs5,13. Additionally, small sample sizes and sampling biases can substantially reduce model accuracy4,8. This is particularly troublesome when attempting to model suitable habitats for a rare species14.
It is also essential that model results are tested for goodness of fit and accuracy before considering the results ecological plausible5. Assessment techniques such as bootstrap, cross-validation, and receiver-operating characteristic plots have been implemented within modelling processes to test the accuracy of model outcomes12.
Recently developed ensemble-forecasting methods (such as the BIOMOD2 platform) can provide a solution to these limitations5. Ensemble models combine the predictions of many different methods and weight the models according to cross-validation, calibration, goodness of fit, and accuracy5,13.
While ensemble modelling might appear to be the answer to all possible limitations, the ability of ensemble models to increase the performance of species distribution models is still debated13,15. But combining and weighting species distribution models in an ensemble approach does not necessarily improve the reliability of the model results, and on occasion, machine-learning methods on their own can provide more reliable and accurate results13,15.
This is likely where model selection (ranking) comes in to play — while ensemble models can produce slightly less reliable results than using a specific modelling method13,15, extensive research is required before an ecologist, conservationist, or environmental scientist will be able to readily select the specific method for their needs. To increase the complexity in selecting the individual models most suited to a specific dataset and intended application, the criteria and recommendations that inform model choice are often incomplete, and are dispersed throughout the literature9.
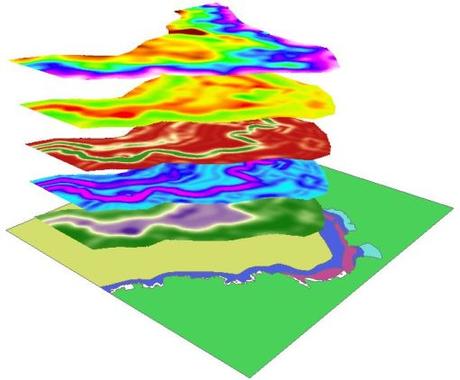
Conclusions
While species distribution models can provide a reliable, cost-effective way of estimating the possible distribution of a species outside of its current range, one still needs to consider that the chosen method(s) suits the dataset and intended application10,12.
The use of species distribution models to guide conservation actions presents a range of limitations; however, many of these limitations are addressed with the use of ensemble approaches5 . While ensemble models might not necessarily be more reliable than individual species distribution-model methods13,15, inexperience and a lack of consensus regarding selection criteria mean that ensemble models still provide a reasonably accurate and reliable method of estimating species distributions9.
With an increase in the development of machine-learning techniques comes new and improved methods of modelling the distributions of species9, bringing with them a new understanding of the factors that drive the distribution of both threatened and pest species alike.
—
- von Humboldt, A. & Bonpland, A. Essai sur la géographie des plantes (1807).
- McPherson, J. M., Jetz, W. & Rogers, D. J. The effects of species’ range sizes on the accuracy of distribution models: ecological phenomenon or statistical artefact? Journal of Applied Ecology 41, 811-823 (2004)
- Pearson, R. G. Species’ distribution modeling for conservation educators and practitioners. Synthesis. American Museum of Natural History 50, 54-89 (2007)
- Stockwell, D. R. & Peterson, A. T. Effects of sample size on accuracy of species distribution models. Ecological Modelling 148, 1-13 (2002)
- Thuiller, W., Lafourcade, B., Engler, R. & Araújo, M. B. BIOMOD–a platform for ensemble forecasting of species distributions. Ecography 32, 369-373 (2009)
- Kearney, M. & Porter, W. Mechanistic niche modelling: combining physiological and spatial data to predict species’ ranges. Ecology Letters 12, 334-350 (2009)
- Elith, J. et al. Novel methods improve prediction of species’ distributions from occurrence data. Ecography 29, 129-151 (2006)
- Bean, W. T., Stafford, R. & Brashares, J. S. The effects of small sample size and sample bias on threshold selection and accuracy assessment of species distribution models. Ecography 35, 250-258 (2012)
- Elith, J. & Graham, C. H. Do they? How do they? WHY do they differ? On finding reasons for differing performances of species distribution models. Ecography 32, 66-77 (2009)
- Elith, J. & Leathwick, J. R. Species distribution models: ecological explanation and prediction across space and time. Annual Review of Ecology, Evolution, and Systematics 40, 677-697 (2009)
- Sutherst, R. W. Pest species distribution modelling: origins and lessons from history. Biological Invasions 16, 239-256 (2014)
- Guisan, A. & Zimmermann, N. E. Predictive habitat distribution models in ecology. Ecological Modelling 135, 147-186 (2000)
- Hao, T., Elith, J., Guillera‐Arroita, G. & Lahoz‐Monfort, J. J. A review of evidence about use and performance of species distribution modelling ensembles like BIOMOD. Diversity and Distributions 25, 839-852 (2019)
- Lomba, A. et al. Overcoming the rare species modelling paradox: a novel hierarchical framework applied to an Iberian endemic plant. Biological Conservation 143, 2647-2657 (2010).
- Hao, T., Elith, J., Lahoz‐Monfort, J. J. & Guillera‐Arroita, G. Testing whether ensemble modelling is advantageous for maximising predictive performance of species distribution models. Ecography 43, 549-558 (2020).