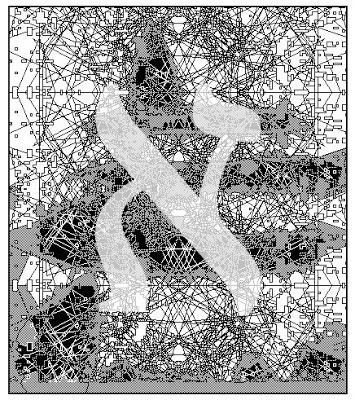
I've published a new working paper. Title above, download links, abstract, table of contents, and introduction below.
Download at:
- academia: https://www.academia.edu/s/9c587aeb25
- SSRN: https://ssrn.com/abstract=3667608
- RaesearchGate: https://www.researchgate.net/publication/343444766_GPT-3_Waterloo_or_Rubicon_Here_be_Dragons
GPT-3 is a significant achievement.
But I fear the community that has created it may, like other communities have done before – machine translation in the mid-1960s, symbolic computing in the mid-1980s, triumphantly walk over the edge of a cliff and find itself standing proudly in mid-air.
This is not necessary and certainly not inevitable.
A great deal has been written about GPTs and transformers more generally, both in the technical literature and in commentary of various levels of sophistication. I have read only a small portion of this. But nothing I have read indicates any interest in the nature of language or mind. That seems relegated to the GPT engine itself. And yet the product of that engine, a language model, is opaque. I believe that, if we are to move to a level of accomplishment beyond what has been exhibited to date, we must understand what that engine is doing so that we may gain control over it. We must think about the nature of language and of the mind.
That is what this working paper sets out to achieve, a beginning point, and only that. By attending to ideas by Adam Neubig, Julian Michael, and Sydney Lamb, and by extending them through the geometric semantics of Peter Gärdenfors, we can create a framework in which to understand language and mind, a framework that is commensurate with the operations of GPT-3. That framework can help us to understand what GPT-3 is doing when it constructs a language model, and thereby to gain control over that model so we can enhance and extend it.
It is in that speculative spirit that I offer the following remarks.
Abstract: GPT-3 is an AI engine that generates text in response to a prompt given to it by a human user. It does not understand the language that it produces, at least not as philosophers understand such things. And yet its output is in many cases astonishingly like human language. How is this possible? Think of the mind as a high-dimensional space of signifieds, that is, meaning-bearing elements. Correlatively, text consists of one-dimensional strings of signifiers, that is, linguistic forms. GPT-3 creates a language model by examining the distances and ordering of signifiers in a collection of text strings and computes over them so as to reverse engineer the trajectories texts take through that space. Peter Gärdenfors’ semantic geometry provides a way of thinking about the dimensionality of mental space and the multiplicity of phenomena in the world, about how mind mirrors the world. Yet artificial systems are limited by the fact that they do not have a sensorimotor system that has evolved over millions of years. They do have inherent limits.
Contents
0. Starting point and preview 1
1. Computers are strange beasts 4
2. No meaning, no how: GPT-3 as Rubicon and Waterloo, a personal view 8
3. The brain, the mind, and GPT-3: Dimensions and conceptual spaces 16
4. Gestalt switch: GPT-3 as a model of the mind 24
5. Engineered intelligence at liberty in the world 26
0. Starting point and preview
GPT-3 is based on distributional semantics. Warren Weaver had the basic idea in his 1949 memorandum, “Translation” (p. 8). Gerard Salton operationalized the idea in his work using vector semantics for document retrieval in the 1960s and 1970s (p. 9). Since then distributional semantics has developed as an empirical discipline. The last decade of work in NLP has seen remarkable, even astonishing, progress. And yet we lack a robust theoretical framework in which we can understand and explain that progress. Such a framework must also indicate the inherent limitations of distributional semantics. This document is a first attempt to outline such a framework, as such its various formulations must be seen as speculative and provisional. I offer them so that others may modify them, replace them, and move beyond them.
It started with a comment at a blog
On July 19, 2020, Tyler Cowen made a post to Marginal Evolution entitled “GPT-3, etc.” It consisted of an email from a reader who asserted, “When future AI textbooks are written, I could easily imagine them citing 2020 or 2021 as years when preliminary AGI first emerged,. This is very different than my own previous personal forecasts for AGI emerging in something like 20-50 years…” While I have my doubts about the concept of AGI – it’s too ill-defined to serve as anything other than a hook on which to hang dreams, anxieties, and fears – I think GPT-3 is worth serious consideration.
Cowen’s post has attracted 52 comments so far, more than a few of acceptable or even high quality. I made a long comment to that post. I then decided to expand that comment into a series of blog posts, say three or four, and then to collect them into a single document as a working paper. When it appeared that those three or four posts would grow to five or six I decided that I would issue two working papers. This first one would concentrate on GPT-3 and the nature of artificial intelligence, or whatever it is. The second would speculate about the future and take a quick tour of the past.
Here is a slightly revised version of the comment I made at Marginal Revolution. This paper covers the shaded material. The rest will be covered in the second paper.
Yes, GPT-3 [may] be a game changer. But to get there from here we need to rethink a lot of things. And where that's going (that is, where I think it best should go) is more than I can do in a comment.While the portion of the comment to be elaborated in the next working paper is considerably longer than the portion being elaborated in this one, I do not expect that paper to be proportionately longer. This paper covered quasi-technical matters requiring fairly careful exposition. The next paper will go by more quickly and will, in sections, approach science fiction.
Right now, we're doing it wrong, headed in the wrong direction. AGI, a really good one, isn't going to be what we're imagining it to be, e.g. the Star Trek computer.
Think AI as platform, not feature (Andreessen). Obvious implication, the basic computer will be an AI-as-platform. Every human will get their own as an very young child. They're grow with it; it’ll grow with them. The child will care for it as with a pet. Hence we have ethical obligations to them. As the child grows, so does the pet – the pet will likely have to migrate to other physical platforms from time to time.
Machine learning was the key breakthrough. Rodney Brooks’ Gengis, with its subsumption architecture, was a key development as well, for it was directed at robots moving about in the world. FWIW Brooks has teamed up with Gary Marcus and they think we need to add some old school symbolic computing into the mix. I think they’re right.
Machines, however, have a hard time learning the natural world as humans do. We're born primed to deal with that world with millions of years of evolutionary history behind us. Machines, alas, are a blank slate.
The native environment for computers is, of course, the computational environment. That's where to apply machine learning. Note that writing code is one of GPT-3's skills.
So, the AGI of the future, let's call it GPT-42, will be looking in two directions, toward the world of computers and toward the human world. It will be learning in both, but in different styles and to different ends. In its interaction with other artificial computational entities GPT-42 is in its native milieu. In its interaction with us, well, we'll necessarily be in the driver’s seat.
Where are we with respect to the hockey stick growth curve? For the last 3/4 quarters of a century, since the end of WWII, we've been moving horizontally, along a plateau, developing tech. GPT-3 is one signal that we've reached the toe of the next curve. But to move up the curve, as I’ve said, we have to rethink the whole shebang.
We're IN the Singularity. Here be dragons.
[Superintelligent computers emerging out of the FOOM is bullshit.]
* * * * *
ADDENDUM: A friend of mine, David Porush, has reminded me that Neal Stephenson has written of such a tutor in The Diamond Age: Or, A Young Lady's Illustrated Primer (1995). I then remembered that I have played the role of such a tutor in real life, The Freedoniad: A Tale of Epic Adventure in which Two BFFs Travel the Universe and End up in Dunkirk, New York.
* * * * *
1. Computers are strange beasts – They’re obviously inanimate, and yet we communicate with them through language. The don’t fit pre-existing (19th century?) conceptual categories, and so we are prone to strange views about them.
2. No meaning, no how: GPT-3 as Rubicon and Waterloo, a personal view – Arguing from first principles it is clear that GPT-3 lacks understanding and access to meaning. And yet it produces very convincing simulacra of understanding. But common sense understanding remains elusive, as it did for old school symbolic processing. Much of common sense is deeply embedded in the physical world. GPT-3, as it currently functions is, in effect, an artificial brain in a vat.
3. The brain, the mind, and GPT-3: Dimensions and conceptual spaces – GPT-3 creates a language model by examining the distances and ordering of signifiers in a collection of text strings and computes over them so as to reverse engineer but the trajectories texts take through a high-dimensional mental space of signifieds. Peter Gärdenfors’ semantic geometry provides a way of thinking about the dimensionality of mental space and the multiplicity of phenomena in the world.
4. Gestalt switch: GPT-3 as a model of the mind – GPT-3 creates: 1) a model of a body of natural language texts, and only a model. 2) Those texts are the product of human minds. 3) Though the application of 2 to 1 we may conclude that GPT-3 is also a model of the mind, albeit a very limited one. 3 requires a Gestalt switch.
5. Engineered intelligence at liberty in the world – The “intelligence” in systems such as GPT-3 is static and reactive. To liberate and mobilize it we need to endow AI systems with mental models of the kind investigated in “old school” symbolic AI.