AI-powered robot is learning to explore the seas. The ocean is vast, and our efforts to comprehend it remain mostly superficial. The National Oceanic and Atmospheric Organization estimate that over 80% of the ocean remains “unmapped, unseen, and unexplored.”
Ships are the major means of gathering information about the oceans, but they are expensive to send out on a regular basis. Argo floats, or robotic buoys, have recently started drifting with the currents, plunging up and down to take a variety of data at depths of up to 6,500 feet. However, new aquatic robots developed by a Caltech lab might rove deeper and perform more customized underwater tasks.
AI-powered robot is learning to explore the seas:
“We’re imagining a global ocean exploration approach in which you take swarms of smaller robots of various types and populate the ocean with them for tracking, climate change, and understanding ocean physics,” says John O. Dabiri, a professor of aeronautics and mechanical engineering at the California Institute of Technology.
CARL-Bot (Caltech Autonomous Reinforcement Learning Robot) arrives at a palm-sized aquatic robot that resembles a pill capsule crossed with a dumbo octopus.
It features motors for swimming,
- The weight to maintain upright,
- And as sensors for pressure, depth, acceleration, and direction.
- Everything CARL performs is powered by an internal microprocessor with a 1-megabyte CPU the size of a postage stamp.
Read more: AI Research Enables Big Data For Detecting Rare Cancer
CARL ocean device:
CARL is the most recent ocean-crossing device to emerge from Dabiri’s lab, and it was designed and 3D-printed at home by Caltech graduate student Peter Gunnarson. Because Caltech’s laboratories were shuttered at the start of 2021 due to COVID, Gunnarson did his first experiments in his bathtub.
CARL can still manage remotely for the time being. But there can’t be any hand-holding to go to the deepest sections of the ocean. That means no researchers directing CARL—it must learn to explore the vast ocean on its own. Gunnarson and Dabiri sought out computer scientist Petros Koumoutsakos, who assisted with the development of AI algorithms for CARL that might educate it to orient itself based on changes in its immediate surroundings and previous experiences. Their findings were published in Nature Communications this week.
CARL may decide to change its course on the fly to avoid the choppy currents and arrive at its target. It may also use “minimum energy” from a lithium-ion battery to stay stationary in a certain spot.
CARL’s strength is based on his recollections:
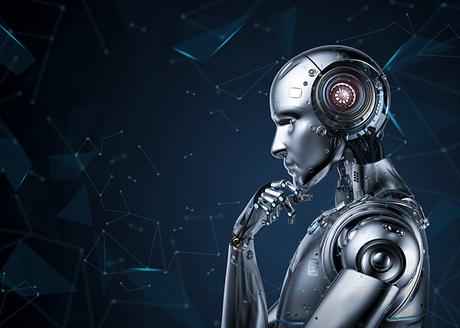
Koumoutsakos’ collection of algorithms can perform wayfinding calculations on board the little robot. The algorithms also make use of the robot’s recollection of previous interactions, such as how to avoid a whirlpool. “We can utilize that information to figure out how to manage those circumstances in the future,” Dabiri says.
CARL’s programming allows it to recall similar courses it has traveled in prior excursions and “become better and better at sampling the water with less time and less energy over repeated encounters,” Gunnarson says.
A large portion of the machine learns to perform in simulation, where all data points are clean. However, bringing that to the actual world might be difficult. Sensors get overload and fail to detect all relevant parameters. “We’re just get start the physical tank,” Gunnarson explains. The initial stage is to see if CARL can perform simple activities like repeated dives. On Caltech’s blog, a brief video shows the robot awkwardly bobbing around and diving into a motionless water tank.
As testing progresses, the team intends to place CARL in a pool-like tank with miniature jets capable of creating horizontal currents for it to maneuver through. When the robot is ready, it will move to a two-story facility that can simulate upwelling and downwelling currents. It will have to find out how to maintain a specific depth in an area where water is flowing in all directions.
“Ultimately, though, we want CARL to exist in the actual world. “He’ll leave the nest and go into the water, and the aim will be for him to learn how to navigate on his own via repeated tries,” Dabiri explains.
Modify by sensors:
The crew will also modify the sensors in and on CARL throughout the experiment. “One of the challenges we have is what is the bare minimum of sensors that can put aboard to done the operation,” Dabiri explains. When a robot is outfitted with instruments such as LiDAR or cameras, “it restricts the system’s ability to travel for very long in the water before you have to change the batteries.”
Researchers might extend CARL’s life by reducing the sensor load and making room for scientific devices that detect pH, salinity, temperature, and other variables.
Read more: Data Visualization Best Practices (2023)
CARL’s software might be the inspiration for the next bionic jellyfish:
- Dabiri’s group released a paper early last year detailing this.
- How they utilized electric zaps to control the motions of a jellyfish.
- It’s likely that adding a chip with machine learning algorithms.
- similar to CARL may allow researchers to better direct the jellyfish across the water.
- How this navigation algorithm works on a real living jellyfish, adds Dabiri.
- CARL serves as a testing platform for the algorithms that may potentially be used in mechanically changed organisms.
- These jellies, unlike robots and rovers not limited by depth.
- Since scientists know they can survive in the Mariana Trench.
- Some 30,000 feet below the surface.
Ocean monitoring:
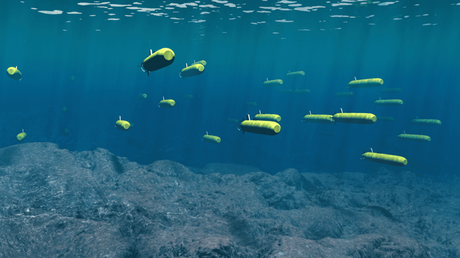
CARL, by itself, can still be a valuable instrument in ocean monitoring. Because it can travel near sea bottoms and other delicate structures.
It can operate with current equipment like Argo floats and embark on solo missions to do more fine-tuned research. It may also track and tag biological species such as a school of fish.
Imagine 10,000 or a million CARLs all going out into the ocean at the same time to measure locations.
That we just can’t access it now so that we can have a time-resolved picture of how the ocean is changing.
“That’s going to be incredibly important not only for climate projections but also for understanding how the ocean operates.”