Discriminatory and filled with institutional bias!
The Dutch childcare benefit scandal has highlighted the need for ideas about how to deal with the use of algorithms. We have chosen Machine Learning (ML) as an interesting Problem Solving Topic for 2022, go here to read more.
Algorithmic decision-making systems are increasingly used to identify and detect people who meet predetermined profiles. Many of these systems have been designed to deal with a particular type of fraud, for example, child benefit fraud. Between 2013 and 2019, Dutch authorities made false accusations and they wrongly accused around 26 000 parents of making fraudulent benefits claims. They required the parents to pay back the money, which in some cases drove the families into severe financial hardships. The working procedures of the Tax and Customs Administration were found by an investigation to be discriminatory and filled with institutional bias and the scandal lead to the resignation of the third Rutte cabinet.
“Our investigation has shown that the Benefits department of the Tax and Customs Administration […] saved and used data in a way that is absolutely prohibited. The whole system was set up in a discriminatory way and was used as such. […] There was permanent and structural unnecessary negative attention for the nationality and dual citizenship of the applicants.” The Dutch Data Protection Authority chairman Aleid Wolfsen
DNA analysis revolutionized the criminal justice system when it first emerged in 1986. Today, gathering DNA evidence is a routine procedure and millions of criminal convictions have been made using this technology. Big data and artificial intelligence have to lead the development of a range of systems and machine learning is one of the most intelligent systems that have been developed. Biometric technologies using the latest machine learning algorithms have led to the development of digital fingerprints, iris recognition and facial recognition. We have a tendency to believe that technology is always effective and does not make errors. This approach and strong belief in technology are of course not great. To minimise the risk of problems, it is necessary to explore strengths and weaknesses with a technology.
What is machine learning?
Machine learning is a form of artificial intelligence (AI) that is based on the idea that systems can learn from data and make decisions with little human intervention. Machines can identify patterns and a system can learn like a human without explicit programming. ML algorithms build a model based on training data and make decisions or predictions based on using this data rather than being programmed to do something. It is used in a range of applications where it is difficult or even unfeasible to develop conventional algorithms to carry out tasks.
Statistical learning is used but also learning that mimics how our biological brain is working. There is an important difference between artificial intelligence and machine learning. AI implies an agent interacting with the environment to learn and take action. In contrast, ML learns and predicts based on passive observations.
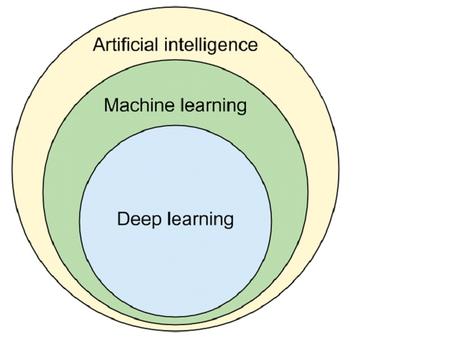
Machine learning as subfield of AI
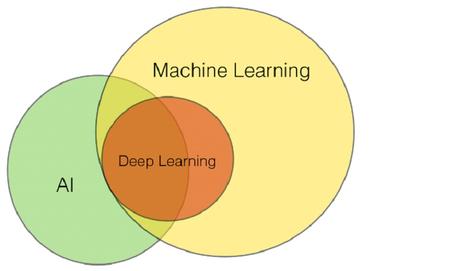
Part of machine learning as subfield of AI or part of AI as subfield of machine learning
The term dataism has been used by Yuval Noah Harari to describe a stage where we trust algorithms and data more than our own logic and judgments. He predicts that eventually, we will give algorithms the authority to make the most important decisions in their lives, for example, which career we should pursue.
Problems with Machine Learning
ML sounds fantastic and great results have been achieved in some areas, yet, there are several issues and it often fails to deliver the expected results. Sometimes ML is the right approach but there are also situations where it simply should not be used – at least not at the moment.
Problems and Limitations
- Misapplication – badly chosen task and algorithms
- Lack of data
- Problem with access to the data
- Data bias
- Privacy problems
- Ethics
- Wrong typ of tools
- Lack of resources
- Problems with evaluation of data
We will continue to explore this fascinating topic in the next blog post.
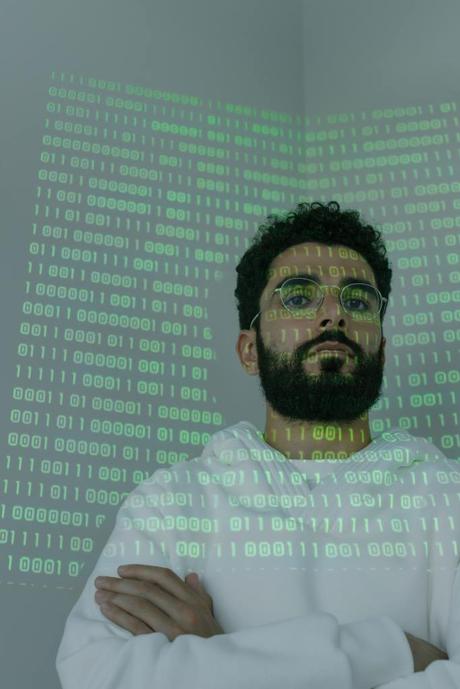